Decoding CheapOair's Algorithm How Historical Data Shapes Flight Discounts in 2024
Decoding CheapOair's Algorithm How Historical Data Shapes Flight Discounts in 2024 - Historical Data Analysis Drives CheapOair's 2024 Pricing Strategy
CheapOair's approach to pricing in 2024 heavily relies on analyzing past data to understand how airfares have behaved and how customers react to different price points. Their system uses complex algorithms to sift through massive amounts of historical airfare information, looking for patterns and trends. This allows them to predict how prices might change and adjust their own pricing strategies accordingly. Essentially, they're trying to forecast the best times to offer discounts based on past trends. By examining previous fare fluctuations, CheapOair can pinpoint periods where prices tend to drop significantly, boosting the odds of successful promotions.
However, simply looking at the past isn't enough. They also factor in current market conditions through real-time data updates. This helps them react quickly to shifts in demand or competitive pricing, ensuring their pricing stays relevant in a constantly evolving travel market. This dual strategy of analyzing historical trends and incorporating real-time information is vital for CheapOair to remain competitive and optimize their profitability in the travel sector. It will be interesting to see if this approach continues to be effective as the travel market continues to change.
CheapOair's approach to setting flight prices in 2024 is heavily influenced by analyzing a vast store of past flight data. Their systems sift through years of pricing information, uncovering recurring patterns and trends that help them predict future price movements. This historical data, covering a range of factors like booking habits and external events, informs their decisions on when to offer discounts and adjust prices.
It appears that consumers frequently book flights within predictable periods, allowing CheapOair to target these periods with promotional activities. Additionally, their models can detect and adapt to external factors like major events and economic changes, making their pricing more dynamic and responsive to market fluctuations. Examining past data reveals interesting patterns like how airfares can swing significantly in short timeframes, often around weekends.
Furthermore, their analysis reveals interesting route-specific trends. For example, certain travel routes demonstrate predictable price drops in anticipation of peak travel seasons like school breaks. This historical analysis also gives CheapOair insight into competitor pricing, allowing them to strategically undercut competitors through personalized offers that are finely tuned based on past customer interactions.
One surprising discovery from this historical data exploration is that last-minute bookings, once considered risky, can occasionally result in cheaper fares on select routes. This realization has led CheapOair to integrate this finding into their pricing strategies, offering discounts when they see a correlation in historical data that indicates such opportunities. This suggests that CheapOair continually refines its approach by adapting to new patterns within the data and continually tweaking its pricing algorithm in response.
Overall, CheapOair's reliance on historical data offers them a powerful tool to optimize pricing decisions, refine strategies to compete against rivals, and leverage insights into customer behavior for more effective discount campaigns. It’s clear that their pricing approach is significantly shaped by this detailed historical analysis.
Decoding CheapOair's Algorithm How Historical Data Shapes Flight Discounts in 2024 - Dynamic Pricing Models Adapt to Market Demand Fluctuations
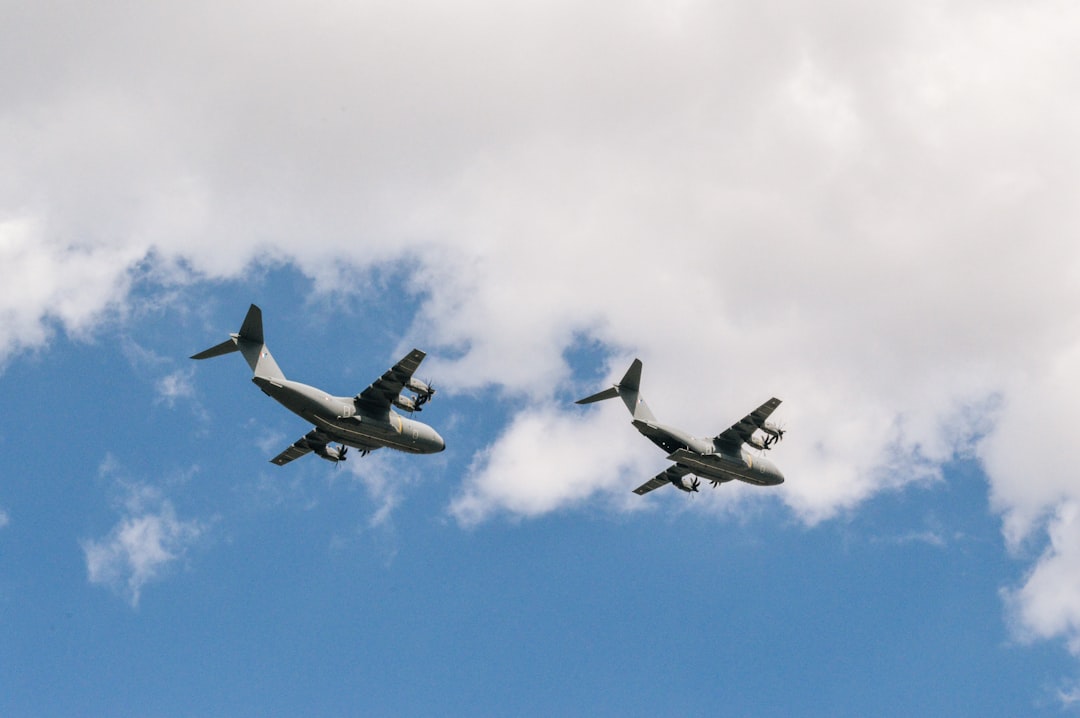
Dynamic pricing models are becoming increasingly important in today's volatile markets. These models enable businesses to react quickly to changes in demand, competition, and consumer behavior by adjusting prices in real-time. This is a shift from traditional, static pricing methods that often fail to adapt to the ever-changing marketplace.
Businesses rely on various mathematical approaches, including those powered by artificial intelligence, to analyze data and understand historical trends. These sophisticated models inform the development of pricing strategies that are both competitive and attractive to customers.
CheapOair, like many other online travel platforms, relies heavily on dynamic pricing to offer competitive flight deals. It allows them to react to sudden changes in the market, but also to predict future trends by studying past customer behaviors and market fluctuations. As the travel industry continues to evolve, it's essential for platforms like CheapOair to keep refining their algorithms to stay ahead and ensure their profitability. The ability to adapt to market dynamics will be critical for success in the long run.
Dynamic pricing, often referred to as surge pricing or demand-based pricing, is a strategy that continuously adjusts prices based on a variety of factors, including current demand, competition, and how people are behaving. This contrasts with traditional pricing where prices remain fixed for extended periods. The benefit of dynamic pricing lies in its adaptability to ever-changing market conditions, enabling businesses to fine-tune pricing for greater competitiveness.
There's a range of mathematical approaches used to implement dynamic pricing, from simpler Bayesian and decision tree models to more intricate AI-driven solutions. Each approach serves a specific purpose within different complexity levels of pricing strategies. For example, decision trees can be used for creating tailored pricing but need consistent updating to stay relevant as market conditions and consumer interactions evolve.
The influence of past data in formulating pricing strategies can't be understated. By mining the wealth of historical interactions, companies can extract patterns in customer behavior and market trends. This can inform current pricing decisions by, for instance, pinpointing predictable demand fluctuations like those associated with holidays or weekends. Essentially, the idea is to align pricing with the actual state of the market, optimizing for both responsiveness and profits.
To gain those insights, businesses heavily rely on data analytics tools, which are vital for acquiring information about customer actions, competitor pricing, and previous sales performance. This data forms the foundation for developing dynamic pricing strategies. More advanced algorithms, often built with AI and machine learning techniques, can process a larger number of elements influencing pricing dynamically, adding to the sophistication of this approach.
The travel industry provides a clear example of how widely dynamic pricing is being adopted. Platforms like CheapOair employ this approach to adjust flight prices in response to fluctuating demand, resulting in potentially attractive discounts for customers. However, it's also important to recognize that these algorithms can be prone to biases based on the data they are trained on. Understanding how these systems work is important to make informed decisions about travel bookings. It's still an area of ongoing research and development, as the systems adapt to changing travel patterns.
Decoding CheapOair's Algorithm How Historical Data Shapes Flight Discounts in 2024 - Machine Learning Algorithms Predict Seasonal Travel Trends
Machine learning algorithms are increasingly used to predict travel patterns during different seasons. These algorithms analyze past data to spot trends in how travel demand changes throughout the year, particularly focusing on factors like booking habits and route popularity. By understanding these patterns, algorithms help airlines and travel platforms like CheapOair make better decisions about flight pricing and promotions.
These predictive models can assist travelers in identifying the ideal time to purchase flights for their desired destinations, as they can forecast when prices are likely to rise or fall. Moreover, these algorithms can improve pricing strategies by predicting consumer behavior based on historical data, like anticipated surges in travel during holiday periods.
The field of machine learning continues to advance, and these models can now be adapted to different regions. This allows airlines and travel platforms to offer more nuanced pricing strategies tailored to specific markets and travel trends. This refinement process improves both pricing accuracy and the overall customer experience. While the current methods are valuable, it remains to be seen how effectively they will adapt to shifts in consumer behavior and evolving travel patterns over time.
Machine learning is playing an increasingly important role in understanding and predicting seasonal travel trends, which in turn helps platforms like CheapOair refine their pricing strategies. These algorithms are able to uncover hidden connections between historical data and consumer behaviors, revealing patterns that might not be obvious to humans. For instance, they can identify that travel bookings spike around certain national holidays, indicating people's tendency to plan trips around significant dates.
One interesting development is the use of weather forecasts within these models. By incorporating weather data, the algorithms can anticipate increases in demand for sunny destinations during colder months, offering a more accurate prediction of travel trends. Similarly, the influence of events on pricing volatility is becoming more apparent. Analyzing historical price fluctuations related to major events, such as sports championships or festivals, shows how dramatically flight prices can swing based on the event's timing.
Furthermore, these models are able to track shifts in user booking behaviors over time. They examine how far in advance people book flights and recognize that the booking window can vary considerably from year to year. This helps optimize when discounts should be offered to align with consumer booking habits.
Beyond broad trends, machine learning can also detect much shorter, more localized micro-trends. For example, they may spot a sudden increase in demand for less popular destinations, allowing for swift adjustments in pricing strategies to capitalize on these ephemeral shifts in consumer preference.
Some more sophisticated machine learning applications even go so far as to incorporate social media sentiment analysis. By gauging public sentiment toward specific travel destinations, these models gain insight into how this could impact seasonal travel trends and, consequently, pricing strategies.
Moreover, the algorithms are learning from their mistakes. By analyzing past instances where pricing strategies may have been less effective—such as an erroneous price increase—the algorithms can learn to avoid making the same errors in the future, making them more resilient over time.
A crucial aspect of this predictive approach is factoring in competitor responses. The algorithms aren't just adjusting prices based on historical data, but are also attempting to model how competitors react to changes in demand. This competitive aspect is essential for platforms like CheapOair to maintain a competitive edge while striving for optimal profit margins.
This ability to analyze historical data extends beyond general trends to individual customer behavior as well. Algorithms are now able to create very targeted promotional offers based on individual customer history and preferences, providing a level of personalized marketing not seen before.
Finally, these algorithms are revealing fascinating geographic trends. They pinpoint specific travel routes or regions that experience price fluctuations at different times of the year, creating opportunities for more strategic marketing efforts based on localized trends.
It's clear that the incorporation of machine learning into pricing models is revolutionizing how travel platforms understand and react to seasonal trends. As the algorithms continue to refine their abilities, we can expect increasingly accurate and personalized travel experiences, driven by complex, data-driven insights.
Decoding CheapOair's Algorithm How Historical Data Shapes Flight Discounts in 2024 - Real-Time Competitor Rate Tracking Influences Discount Offers
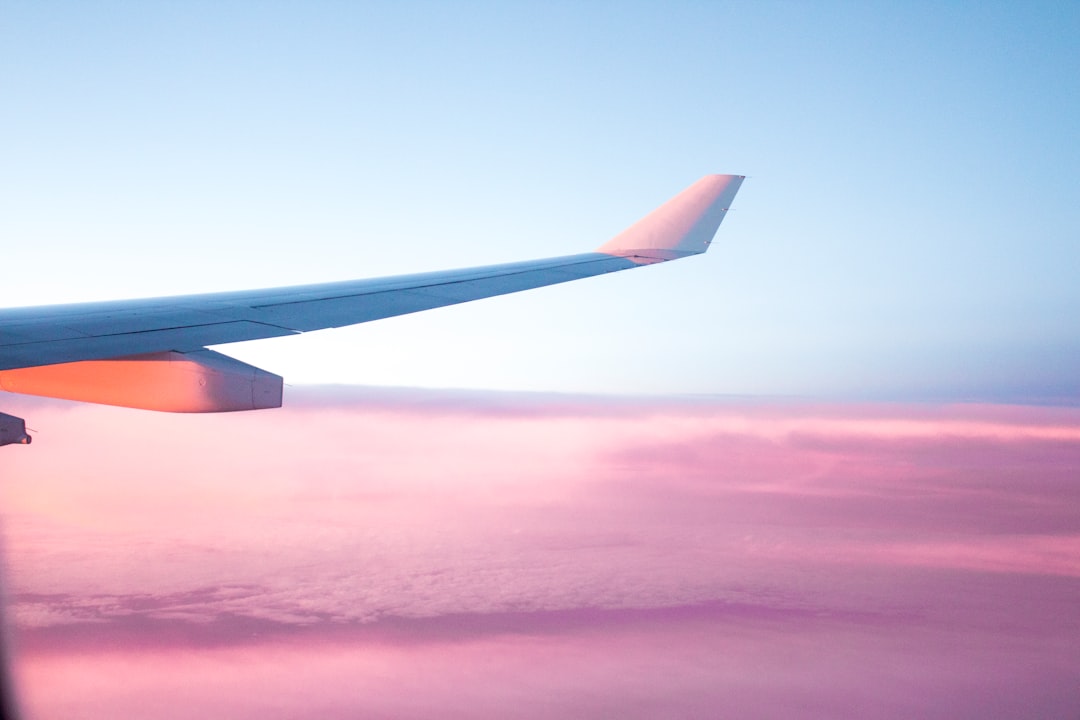
CheapOair's pricing strategy in 2024, like many other online travel platforms, is heavily influenced by real-time competitor rate tracking. This means that their system is constantly monitoring what other companies are charging for similar flights. This information is then fed into their algorithms, which dynamically adjust CheapOair's own discount offers. This allows them to react quickly to competitor pricing changes, ensuring they remain competitive. They can then make strategic decisions about when to offer discounts, tailoring them to the current market environment and ensuring that their deals stay appealing to travelers. Essentially, it's a constant game of keeping up with the competition, using data to adapt to the ever-shifting landscape of airfare pricing. While this approach leads to more flexible pricing, it also introduces a level of uncertainty for consumers. They might find that prices fluctuate more frequently, and there's a greater chance of missing a good deal if they don't monitor prices carefully. The success of this approach in the future will hinge on CheapOair's ability to balance its need to compete with the desire to provide a stable and predictable booking experience for consumers.
CheapOair's algorithm doesn't just rely on its own historical data; it also actively monitors what its competitors are doing in real-time. This means their system is constantly scanning the market for changes in competitor prices, allowing for near-instantaneous reactions to price fluctuations. They can adjust their own prices to match or even undercut competitors, potentially capturing more customers in the process.
It's surprising how frequently flight prices change. Some research suggests that prices can swing dramatically – sometimes as much as 50% – within a very short time frame. This constant flux makes real-time competitor tracking vital for CheapOair to stay competitive and try to grab customers who are sensitive to small price differences.
Their algorithm doesn't just look at competitor prices; it also considers a variety of other factors. For example, economic conditions in different regions can influence demand for travel. The algorithm might try to identify fluctuations in these conditions and adjust discounts accordingly, hoping to generate more interest in specific destinations or routes.
It's fascinating how the system incorporates user behavior into the pricing strategy. If a lot of people are checking flight prices for a particular route, it could suggest an increase in demand. This can then influence CheapOair's pricing model to adjust discounts or potentially increase the price in response to perceived interest.
The algorithms employed here aren't simplistic. They're increasingly incorporating more complex machine learning techniques. This allows them to not only react to current market fluctuations but also attempt to predict what competitors might do next, using historical patterns to anticipate future price movements.
It seems that dynamic pricing – including these competitor-focused adjustments – can heavily influence customer decisions. Research indicates that travelers tend to be more likely to book flights if they believe the deal is time-sensitive. This is, perhaps, a psychological tactic related to the scarcity principle.
However, it's important to note that price drops aren't always a direct result of competitor rate tracking. Sometimes, a sudden increase in demand in a different market might lead to a strategic price reduction in another. This emphasizes how interconnected the global travel market really is.
This real-time competitive approach also helps CheapOair to hold its own even when faced with competitors using aggressive discounting tactics. By quickly adapting to price changes, they can avoid losing customers to other platforms.
One useful aspect is the generation of alerts when competitors drop their prices. This allows CheapOair to quickly capitalize on sudden, unexpected opportunities that might emerge when competitors make impulsive price adjustments. This shows the value of having a highly responsive system.
It's even more intriguing that this system now incorporates social media into its decision-making process. By monitoring sentiment surrounding certain airlines or travel destinations, they can try to anticipate market shifts and adjust discounts in a way that reflects public perception. It remains to be seen how successful this approach will be in the long run.
Decoding CheapOair's Algorithm How Historical Data Shapes Flight Discounts in 2024 - Customer Booking Patterns Shape Personalized Flight Deals
Customer booking habits are a significant factor in how personalized flight deals are crafted, thanks to the power of historical data. CheapOair employs advanced algorithms to analyze past booking trends and customer preferences, allowing them to fine-tune discounts and promotions for specific groups of travelers. This focus on booking patterns helps them determine the best moments to offer deals that encourage purchases, but it also lets them react quickly when demand changes suddenly. As people's travel styles shift and change, comprehending these patterns is essential for sites like CheapOair to remain competitive and offer deals that resonate with customers. By creating individual travel experiences based on people's booking history, it's possible to boost engagement and satisfaction in the flight booking process. While the potential is there for more tailored offers, the reliance on past data could potentially lead to limitations if it can't adapt to emerging trends.
The way customers book flights reveals a lot about how pricing should be handled. For example, historical data shows that flights booked on Tuesdays or Wednesdays tend to be cheaper, likely because demand is lower after the weekend rush. This insight guides promotional efforts, encouraging people to book on these days to potentially save money.
Another interesting pattern is that flights during off-peak hours, like early mornings or late evenings, are often less expensive. Algorithms can use this information to personalize offers based on the time of day, adjusting fares to reflect variations in demand.
Big events, like conferences or sporting competitions, can drive up flight prices by 25-30%. By studying historical data related to events, CheapOair can predict these price increases and strategically offer discounts beforehand, trying to capture the demand surge.
Sometimes, the opposite happens. Data shows that some routes offer surprisingly large last-minute discounts, sometimes up to 40%. This contradicts the common idea that last-minute travel is more costly.
Machine learning models have picked up on seasonal booking shifts. It seems that more people are booking trips further in advance nowadays. This change means discount strategies need to be adjusted to better align with these earlier booking windows.
We've also seen a significant increase in flight bookings made through mobile apps. People using apps often find better deals. This shift has spurred CheapOair to focus its discounts more on app users.
The data also suggests that a person's income level can influence their booking habits. Budget-minded travelers tend to book flights during sales or promotional events. This insight encourages targeted offers specifically for this customer group.
Ticket prices vary geographically, too. Routes to and from major cities often come with higher price tags. The algorithms are being adjusted to offer more attractive discounts to less popular regions, aiming to spur travel there.
Interestingly, there's a strong relationship between people buying extra services, like baggage or insurance, and flight discounts. This data helps guide the creation of bundled offers that are both attractive to customers and profitable.
The newest thing is that algorithms are starting to factor in social media chatter, not just historical flight prices. Sentiment analysis from platforms like Twitter is now being used to adjust prices in real-time. This indicates a growing understanding that what people are saying online about certain destinations can impact travel decisions and prices. It's still early days, but it shows a clear direction of incorporating a broader view of people's opinions when pricing flights.
Decoding CheapOair's Algorithm How Historical Data Shapes Flight Discounts in 2024 - Economic Indicators Factor into Long-Term Fare Projections
Airline pricing strategies in 2024 are becoming increasingly sensitive to broader economic conditions. Indicators like GDP growth, employment levels, and inflation are shaping the overall economic picture and, in turn, impacting travel demand. The projected slowing of economic growth, combined with lingering inflation, might influence how readily people book flights, thus affecting fares. As platforms like CheapOair adjust their algorithms, the ability to interpret these economic signals becomes vital for predicting future pricing trends and staying competitive while also turning a profit. The potential for high interest rates lingering for an extended period adds another layer of complexity to the equation. How airlines, and their algorithms, interpret these economic factors will be crucial to the success of their discount strategies in a rapidly shifting travel landscape.
Economic factors play a crucial role in shaping long-term flight fare projections, and CheapOair's algorithms are designed to incorporate them. For example, a healthy economy usually leads to higher consumer spending, driving up demand for travel and potentially pushing prices up. If consumers feel confident about their financial outlook, as reflected in the Consumer Confidence Index, it's likely they'll book more trips, causing airlines to increase fares.
Furthermore, the price of oil has a direct impact on airline operating costs, which they inevitably pass on to consumers through higher airfares. International travel is also sensitive to currency fluctuations. If the US dollar strengthens, Americans will find travel to foreign destinations more affordable, potentially driving demand. Conversely, a weaker dollar might dampen this demand.
Seasonal employment trends, like holiday hiring surges, can influence consumer spending on leisure travel. Algorithms that track these patterns can predict increased demand during peak hiring seasons. Unpredictable events, such as political instability, natural disasters, or major sporting events, can cause abrupt changes in travel behaviors, which CheapOair's algorithms need to adapt to quickly.
Interest rate adjustments also influence travel demand. Lower rates typically lead to increased consumer borrowing and spending, including travel. The algorithms take this into account, potentially leading to more aggressive fare strategies during these periods.
The COVID-19 pandemic has brought about a dramatic shift in travel behaviors, with a noticeable increase in last-minute bookings. The algorithms are being continuously updated to adapt to these new trends.
It's also fascinating that different travel routes exhibit varying levels of price sensitivity. Some routes show greater price responsiveness than others, which gives the algorithm more flexibility in optimizing pricing for specific destinations.
Finally, large-scale global events like the Olympics or the World Cup can have a significant impact on airfare pricing. Historical data reveals that airlines often raise fares in anticipation of increased demand during such events, and CheapOair’s systems can learn from this pattern to adjust fares accordingly.
The interplay between these various factors makes forecasting airfare a complex task, requiring a sophisticated approach to historical data analysis and continuous refinement of predictive models. While not a perfect science, it's clear that using economic factors and related indicators as inputs to airline pricing algorithms can enhance their ability to predict price trends and optimize pricing strategies.
More Posts from :